Driver Guidance System (DGS) for Taxi Drivers
DGS enables taxi drivers to be more productive by digesting real-time demands and competitions.
Background
Uber and Uber-like services have been rocking the taxi industry globally for the past few years. Traditional taxi companies have been slow in responding to these challenges. The development of the DGS technology (Jha et al., 2018; Jha et al., 2018) aims to help taxi drivers to compete against ride-hailing technologies by providing guidance to an area with predicted demand (i.e., actionable driving decision) that would help drivers shorten their vacant cruising time before finding the next passenger.
The key enabling technology behind the development of the DGS is the stream data processing, demand prediction (Cheng & Rathnayaka, 2025; Cheng & Rathnayaka, 2023), and personalized decision support system (Varakantham et al., 2012; Ahmed et al., 2012).
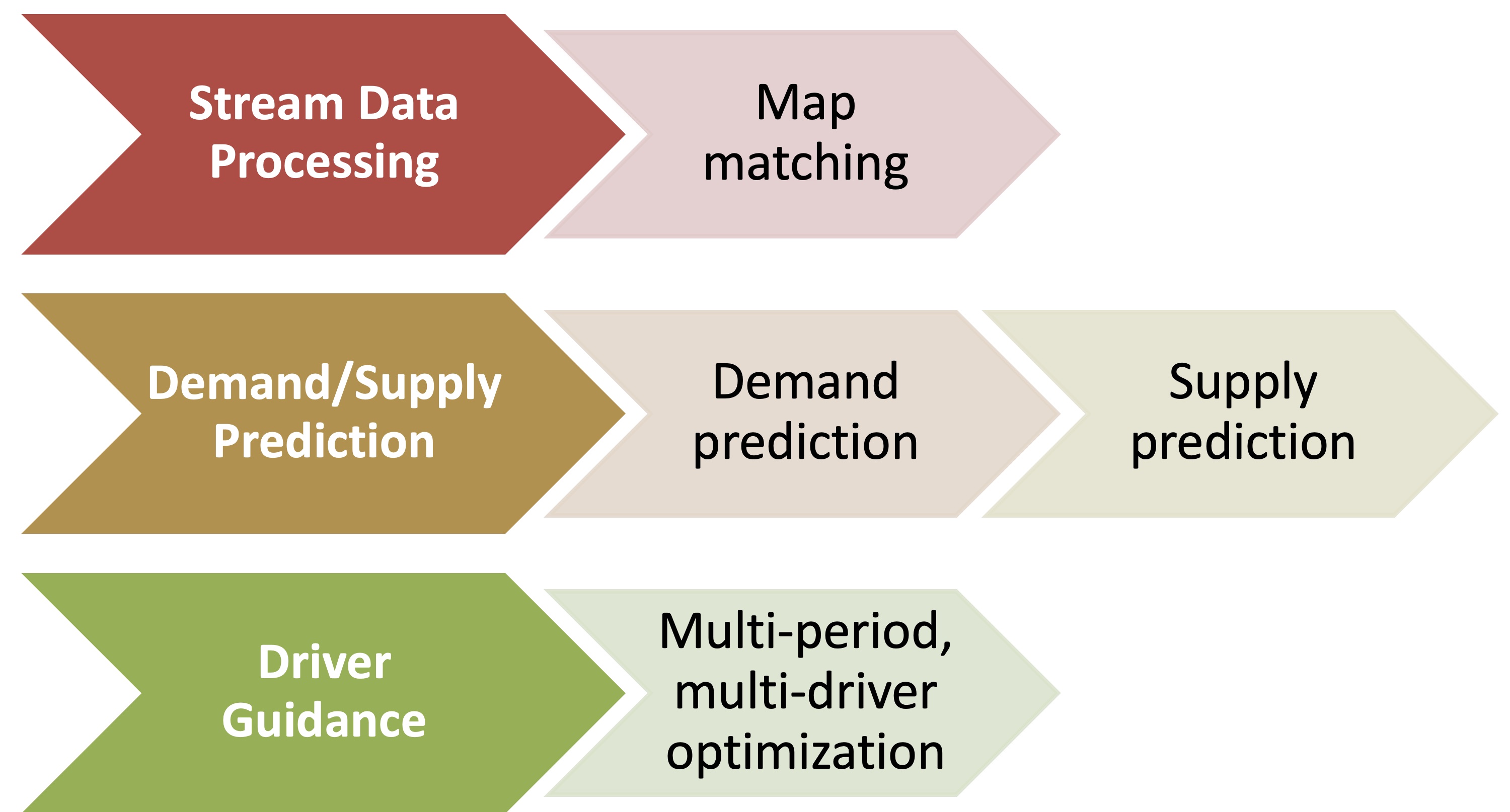
The DGS App
The technology is delivered via a smartphone App. The DGS App is designed to be hands-free; as drivers drive around, the App will automatically provide the best recommendations for individual drivers depending on their respective locations.
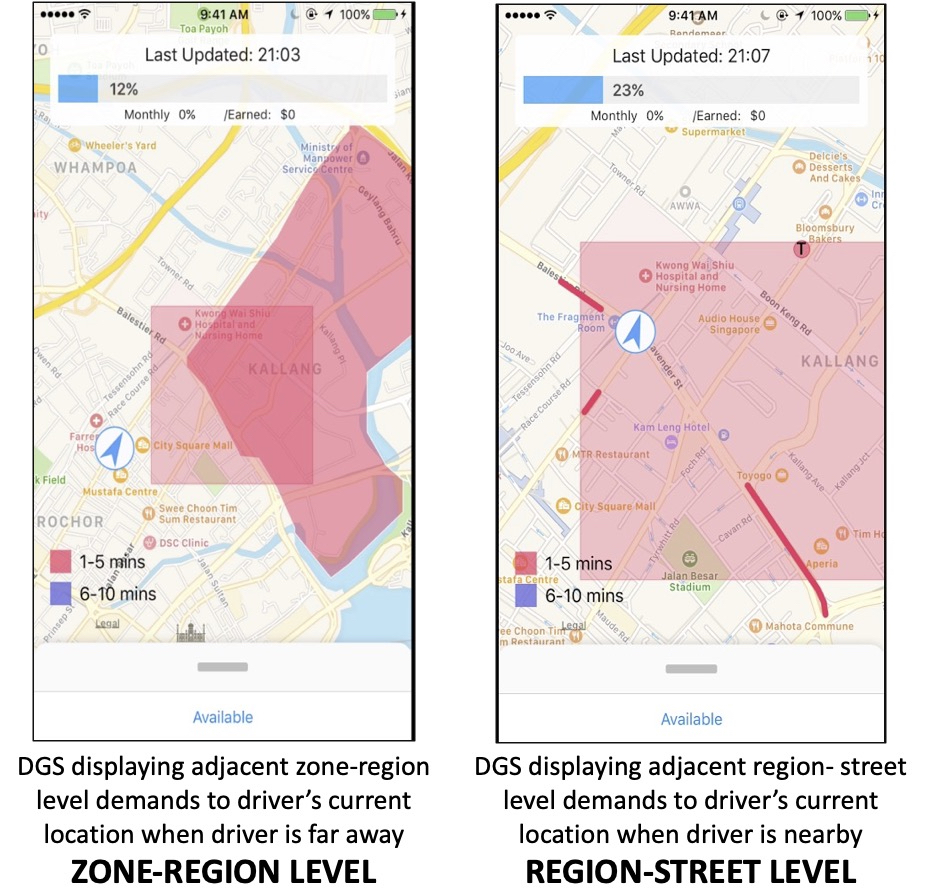
Simulated Performance Evaluation
The expected performance of DGS App is evaluated a massive scale agent-based simulation (Cheng & Nguyen, 2011). As shown in Figure 3 below, the personalized guidance ensures that DGS stays competitive even when the adoption ratio increases.
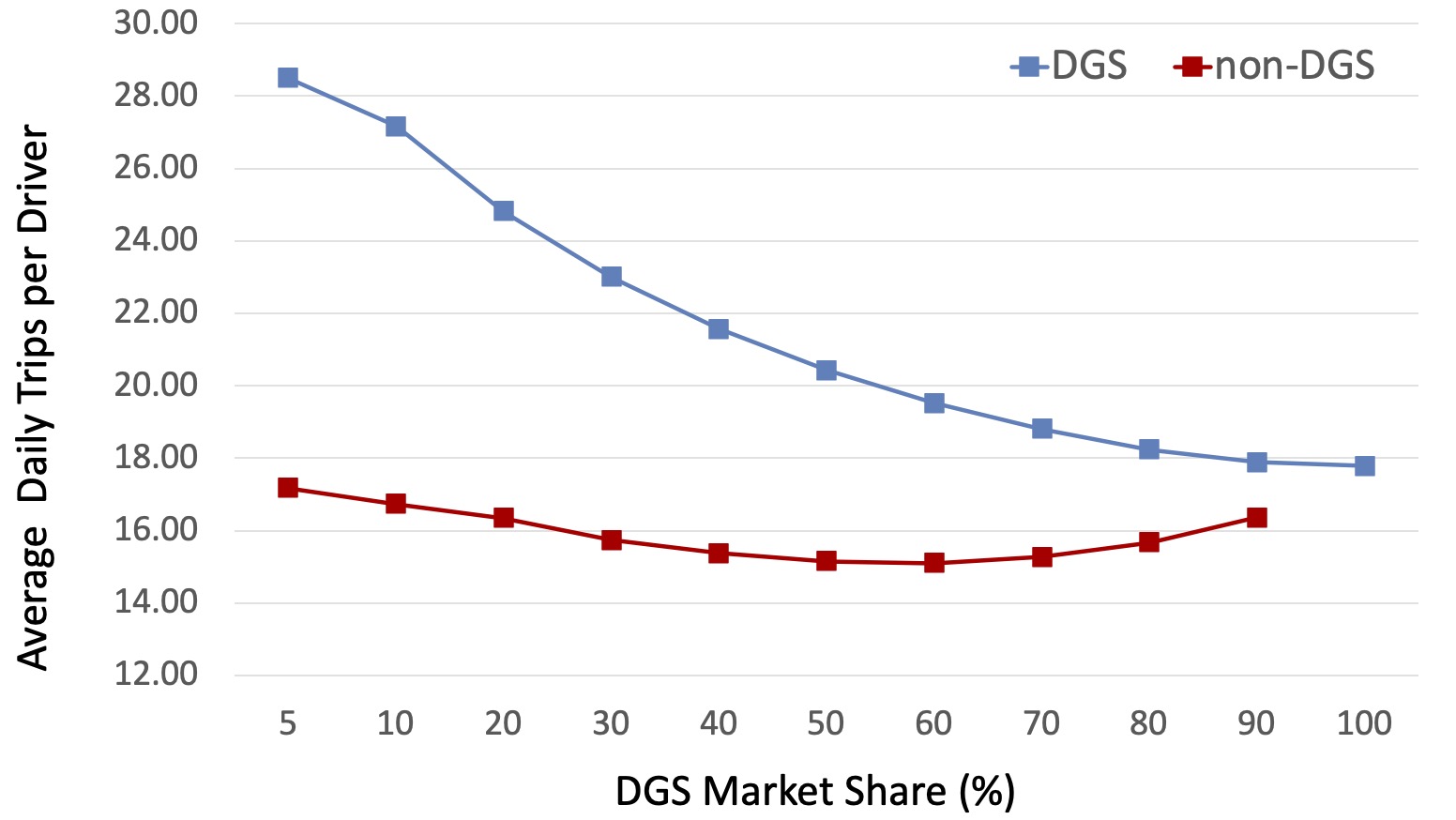
Field Trial Results
The DGS is field tested in Singapore from 2017 to 2018. In total we have recruited 500 taxi drivers to try DGS. On average, following DGS guidance results in 34% reduction in vacant roaming time (Cheng et al., 2018).
The reduction in the vacant roaming time is particularly evident when the demand levels are low (e.g., from 11pm to 5am in Figure 4). We also track the performance of DGS across Singapore, and the improvements are universal (Figure 5).
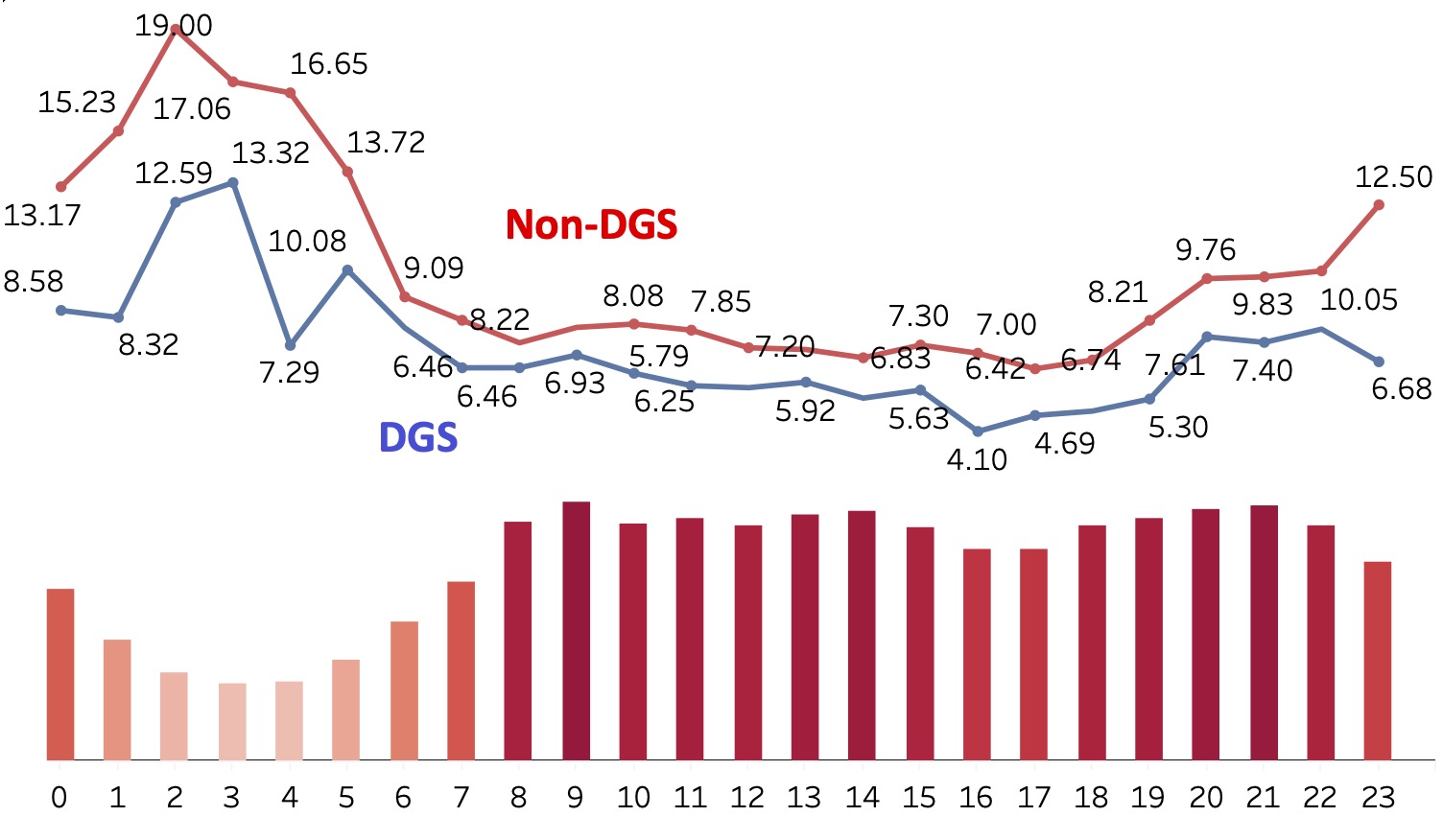
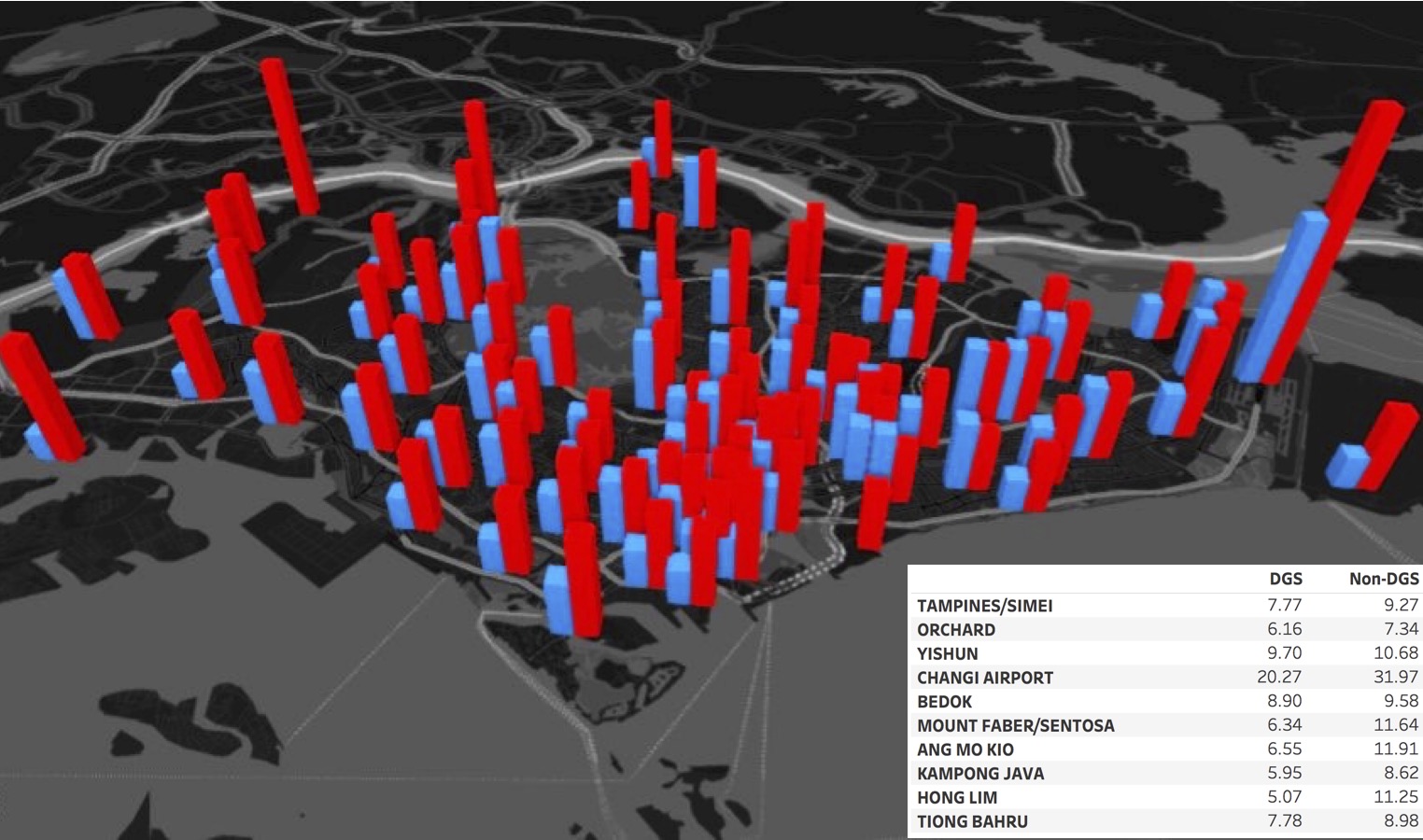
Finally, we also breakdown the performance of DGS by demand channels such as “street hail” or “booking”. We can see that while the booking channel indeed helps drivers in reducing their vacant roaming time (from ~13min to 9min), having personalized guidance still helps in further bringing down the vacant roaming time by ~24%.
Furthermore, after accounting for the “response time” (i.e., the time between driver’s commitment to the job and the passenger pick-up), we can see that the street-hail channel with DGS can be even more efficient than the booking channel (35% vacant vs. 43% vacant).
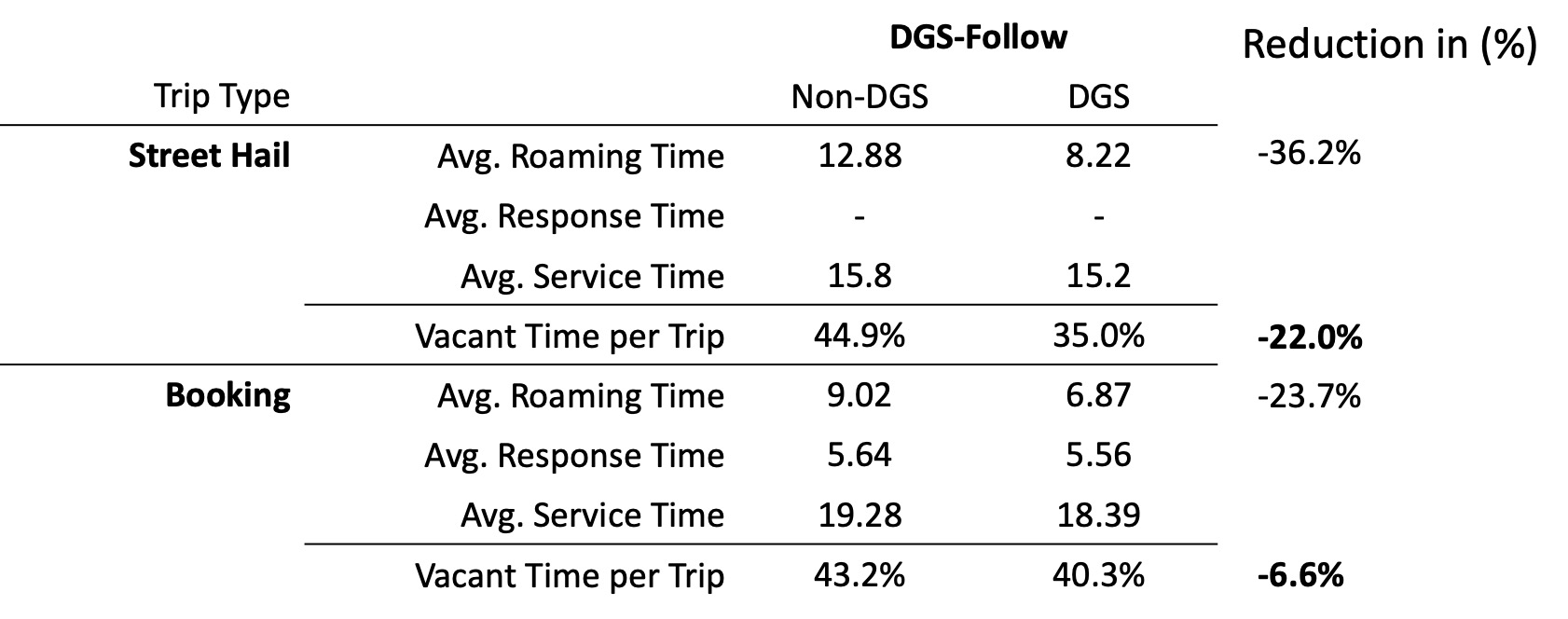
The DGS has also been commercially tested in Tokyo, Japan in April 2020. With dedicated taxi drivers hired to follow DGS exclusively, we observe that DGS-guided drivers experienced 12% less vacant time than non-DGS drivers.
References
2025
-
PatentMethod and System for Taxi Demand Prediction Using a Neural Network ModelSingapore Patent 10202103115Q, Mar 2025
2023
2018
-
AAMAS-18A driver guidance system for taxis in SingaporeIn Seventeenth International Conference on Autonomous Agents and Multiagent Systems, May 2018